Kaplan Meier
Contents
Kaplan Meier#
Core idea start with haz function and go backwards sample->haz->surv->cdf->pmf.
Imports#
import os
import pandas as pd
import numpy as np
import scipy as sp
import matplotlib.pyplot as plt
import seaborn as sns
from scipy.stats import gaussian_kde
sns.set()
Dog Adoption Data#
Investigating time it takes to get dog adopted from a shelter
Every 10 weeks visit shelter
Note: Arrival time of each dog and adoption time of each job
obs = pd.DataFrame()
obs['Start'] = 0,1,2,2,4,6,7
obs['End'] = 5,2,6,9,9,8,9
obs['Status']= 1,1,1,0,0,1,0 # 1 -> Adopted, 0 -> Not adopted
obs
Start | End | Status | |
---|---|---|---|
0 | 0 | 5 | 1 |
1 | 1 | 2 | 1 |
2 | 2 | 6 | 1 |
3 | 2 | 9 | 0 |
4 | 4 | 9 | 0 |
5 | 6 | 8 | 1 |
6 | 7 | 9 | 0 |
Potting Lifelines#
def plot_lifelines(df):
fig, ax = plt.subplots(1, figsize=(12,6))
for i, row in df.iterrows():
if row['Status']==0: plt.hlines(i,row['Start'], row['End'], color='C0') # complete
else: #incomplete
plt.hlines(i, row['Start'], row['End'], color='C1')
plt.plot(row['End'], i, marker='o', color='C1')
plt.xlabel('Time (weeks)')
plt.ylabel('Dog index')
plt.gca().invert_yaxis()
plot_lifelines(obs)
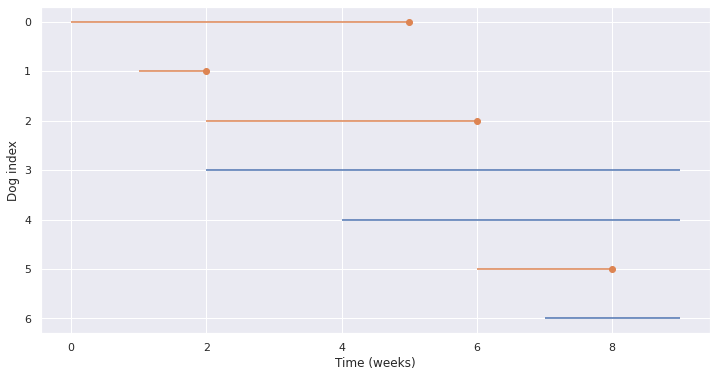
duration = obs['End'] - obs['Start']
Reverse Calculation#
Key Ideas
Shift the lifetimes as if everything started at zero
Compute hazard rate by considering
How many dogs were there which could have been adopted at each time stamp
How many were actually adopted at any particular timestamp
shifted = obs.copy()
shifted['Start'] = 0
shifted['End'] = duration
plot_lifelines(shifted)
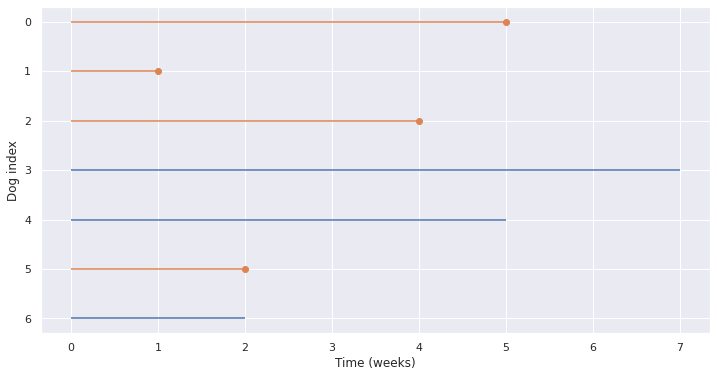
complete = duration[obs['Status']==1]; complete
ongoing = duration[obs['Status']==0]; ongoing
3 7
4 5
6 2
dtype: int64
complete
0 5
1 1
2 4
5 2
dtype: int64
ongoing
3 7
4 5
6 2
dtype: int64
def make_pmf(sample):
return pd.Series(sample).value_counts().sort_index()
make_pmf(complete)
1 1
2 1
4 1
5 1
dtype: int64
make_pmf(ongoing)
2 1
5 1
7 1
dtype: int64
df = pd.DataFrame(dict(pmf_complete=make_pmf(complete), pmf_ongoing=make_pmf(ongoing))).fillna(0); df
pmf_complete | pmf_ongoing | |
---|---|---|
1 | 1.0 | 0.0 |
2 | 1.0 | 1.0 |
4 | 1.0 | 0.0 |
5 | 1.0 | 1.0 |
7 | 0.0 | 1.0 |
df.loc[:,['s_complete', 's_ongoing']]=(df.sum()-df.cumsum()).values # survival function ->dogs surviving
df['at_risk'] = df.sum(axis=1)
df
pmf_complete | pmf_ongoing | s_complete | s_ongoing | at_risk | |
---|---|---|---|---|---|
1 | 1.0 | 0.0 | 3.0 | 3.0 | 7.0 |
2 | 1.0 | 1.0 | 2.0 | 2.0 | 6.0 |
4 | 1.0 | 0.0 | 1.0 | 2.0 | 4.0 |
5 | 1.0 | 1.0 | 0.0 | 1.0 | 3.0 |
7 | 0.0 | 1.0 | 0.0 | 0.0 | 1.0 |
df['hazard'] = df['pmf_complete']/df['at_risk']; df
pmf_complete | pmf_ongoing | s_complete | s_ongoing | at_risk | hazard | |
---|---|---|---|---|---|---|
1 | 1.0 | 0.0 | 3.0 | 3.0 | 7.0 | 0.142857 |
2 | 1.0 | 1.0 | 2.0 | 2.0 | 6.0 | 0.166667 |
4 | 1.0 | 0.0 | 1.0 | 2.0 | 4.0 | 0.250000 |
5 | 1.0 | 1.0 | 0.0 | 1.0 | 3.0 | 0.333333 |
7 | 0.0 | 1.0 | 0.0 | 0.0 | 1.0 | 0.000000 |
df['surv'] = (1 - df['hazard']).cumprod(); df # What are we doing ?
pmf_complete | pmf_ongoing | s_complete | s_ongoing | at_risk | hazard | surv | |
---|---|---|---|---|---|---|---|
1 | 1.0 | 0.0 | 3.0 | 3.0 | 7.0 | 0.142857 | 0.857143 |
2 | 1.0 | 1.0 | 2.0 | 2.0 | 6.0 | 0.166667 | 0.714286 |
4 | 1.0 | 0.0 | 1.0 | 2.0 | 4.0 | 0.250000 | 0.535714 |
5 | 1.0 | 1.0 | 0.0 | 1.0 | 3.0 | 0.333333 | 0.357143 |
7 | 0.0 | 1.0 | 0.0 | 0.0 | 1.0 | 0.000000 | 0.357143 |
Hazard Function: If I am alive at time t what’s the probability of dying at time t. Here, if I am at kennel in time t , what’s the probability of being adopted
Completment - probability of not being adopted
Probabiliy of not being adopted at time t=0, then t=1… This will be product (becoz we are in probabilities)
Survival : cumprod
df['cdf'] = 1- df['surv']; df
pmf_complete | pmf_ongoing | s_complete | s_ongoing | at_risk | hazard | surv | cdf | |
---|---|---|---|---|---|---|---|---|
1 | 1.0 | 0.0 | 3.0 | 3.0 | 7.0 | 0.142857 | 0.857143 | 0.142857 |
2 | 1.0 | 1.0 | 2.0 | 2.0 | 6.0 | 0.166667 | 0.714286 | 0.285714 |
4 | 1.0 | 0.0 | 1.0 | 2.0 | 4.0 | 0.250000 | 0.535714 | 0.464286 |
5 | 1.0 | 1.0 | 0.0 | 1.0 | 3.0 | 0.333333 | 0.357143 | 0.642857 |
7 | 0.0 | 1.0 | 0.0 | 0.0 | 1.0 | 0.000000 | 0.357143 | 0.642857 |
df['pmf'] = np.diff(df['cdf'], prepend=0); df
pmf_complete | pmf_ongoing | s_complete | s_ongoing | at_risk | hazard | surv | cdf | pmf | |
---|---|---|---|---|---|---|---|---|---|
1 | 1.0 | 0.0 | 3.0 | 3.0 | 7.0 | 0.142857 | 0.857143 | 0.142857 | 0.142857 |
2 | 1.0 | 1.0 | 2.0 | 2.0 | 6.0 | 0.166667 | 0.714286 | 0.285714 | 0.142857 |
4 | 1.0 | 0.0 | 1.0 | 2.0 | 4.0 | 0.250000 | 0.535714 | 0.464286 | 0.178571 |
5 | 1.0 | 1.0 | 0.0 | 1.0 | 3.0 | 0.333333 | 0.357143 | 0.642857 | 0.178571 |
7 | 0.0 | 1.0 | 0.0 | 0.0 | 1.0 | 0.000000 | 0.357143 | 0.642857 | 0.000000 |